How AI and Machine Learning are Shaping Production Lines in 2025
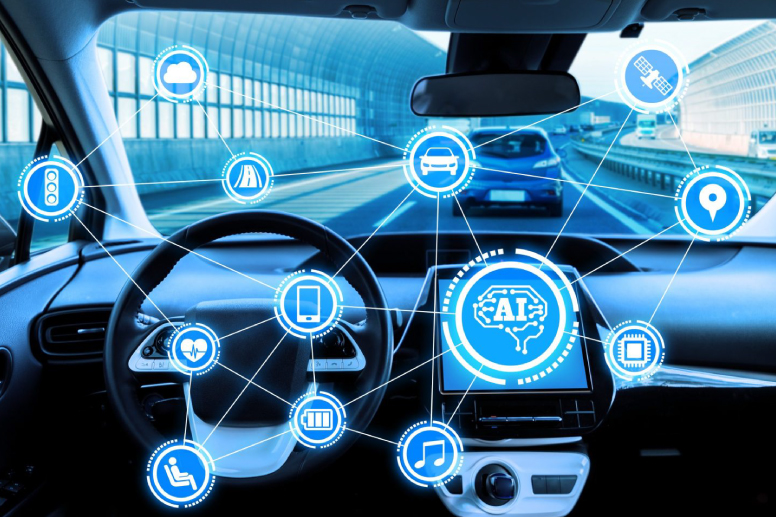
How AI and Machine Learning are Shaping Production Lines in 2025
AI and machine learning are reshaping production systems, offering solutions beyond traditional automation to create intelligent, adaptive manufacturing environments.
These technologies bring unparalleled efficiency, precision, and agility to production lines, allowing manufacturers to remain aggressive in a market that demands rapid adaptability and flawless execution. With tools like predictive maintenance, real-time quality control, and data-driven decision-making, AI empowers manufacturers to reduce costs, minimize waste, and deliver scale-quality products.
What is AI in Production?
Artificial intelligence (AI) in production refers to the application of intelligent systems and algorithms designed to optimize manufacturing processes, improve decision-making, and enhance operational efficiency. These technologies leverage advanced capabilities such as machine learning, predictive analytics, and automation to address key challenges in manufacturing environments. AI enables manufacturers to identify patterns, predict outcomes, and respond proactively to issues, resulting in increased precision, minimized waste, and faster adaptation to shifting market demands.
Unlike traditional automation, AI in production introduces systems capable of learning from historical data, adapting to new scenarios, and making proactive adjustments in real time. This means manufacturers can predict customer demand, detect and prevent equipment failures, and fine-tune inventory management with unprecedented accuracy. As a result, AI empowers businesses to maintain consistent quality, reduce costs, and enhance the flexibility of production lines.
The integration of AI and machine learning in production lines has become essential for manufacturers aiming to remain agile. From improving forecasting to optimizing maintenance schedules, AI-based tools deliver actionable insights and measurable results. These advancements mark a significant evolution in how manufacturing is managed, ensuring operations stay efficient and responsive.
Benefits of Implementing AI in Production
“AI-driven systems analyze vast amounts of production data to identify inefficiencies and improve workflows, maximizing productivity and reducing errors.”
Integrating artificial intelligence into production systems delivers significant advantages for manufacturers seeking to enhance efficiency, maintain consistent quality, and reduce operational costs. AI transforms traditional manufacturing by providing actionable insights, improving decision-making, and automating repetitive tasks. These capabilities enable manufacturers to meet customer demands, optimize resource allocation.
AI systems are particularly effective in addressing inefficiencies, preventing downtime, and scaling operations without sacrificing quality. Below are the most impactful benefits of AI in production, showcasing how this technology is shaping the future of manufacturing.
- Enhanced Process Optimization: AI systems analyze vast production data to detect inefficiencies, minimize errors, and improve productivity. Advanced algorithms dynamically adjust workflows, reduce cycle times, and optimize resource utilization, resulting in streamlined operations.
- Improved Quality Control: AI-powered tools such as computer vision and predictive analytics monitor production processes and identify defects early. This ensures consistent quality, reduces waste, and minimizes rework, enhancing customer satisfaction and strengthening product reliability.
- Predictive Maintenance Capabilities: AI leverages equipment performance data to anticipate potential failures before they occur. This proactive maintenance approach minimizes costly downtime, extends machinery lifespan, and reduces repair expenses, allowing teams to focus on strategic priorities.
- Lower Operational Costs: Automating repetitive and labor-intensive tasks reduces reliance on manual labor, enabling manufacturers to allocate human resources to higher-value activities. AI also improves energy efficiency and materials usage, leading to significant cost savings across the operation.
- Faster Time-to-Market: AI systems accelerate production agility by adapting to fluctuating market demands and streamlining design and testing processes. Manufacturers can bring new products to market more efficiently while maintaining consistent quality and delivery standards.
- Sustainability Improvements: AI supports resource optimization by reducing waste and energy consumption. These enhancements promote environmentally sustainable manufacturing practices while meeting growing consumer and regulatory expectations.
AI production tools change traditional manufacturing operations into intelligent, agile systems that respond to challenges. From improving efficiency and reducing costs to delivering exceptional product quality, the benefits of AI integration create lasting value and scalability for manufacturers. These tools help align production capabilities with long-term business objectives, driving sustainable growth and market leadership.
Understanding Machine Learning in Production
“Machine learning algorithms in production identify patterns, predict outcomes, and optimize operations in real-time, allowing smarter manufacturing processes.”
Machine learning in production involves using algorithms and data-driven models to improve efficiency and accuracy across manufacturing processes. Unlike traditional programming, machine learning allows systems to learn from historical data and improve their performance over time without requiring explicit instructions. This ability makes it a valuable tool for tackling complex challenges in production environments.
Machine learning algorithms in production are used to identify patterns, predict outcomes, and optimize operations in real time. For instance, supervised learning models can classify defective products by analyzing size, shape, or colour features. In contrast, unsupervised learning models can detect anomalies in equipment performance that indicate potential failures. Reinforcement learning, another branch of machine learning, can refine robotic processes through trial-and-error simulations, allowing machines to continuously adapt and improve their tasks.
Integrating machine learning into production gives manufacturers predictive maintenance, request forecasting, and process optimization capabilities. These technologies allow production systems to operate more efficiently, reduce waste, and respond quickly to shifts in market requests. The adaptability of machine learning ensures that manufacturers can stay ahead in an environment where precision and speed are critical for success.
6 Steps to Deploying Machine Learning Models in Production
Deploying machine learning models in production transforms data insights into actionable results, allowing businesses to optimize processes and improve decision-making. A successful deployment strategy ensures these models operate seamlessly, providing real-time predictions and long-term scalability. Machine learning models can drive innovation, reduce inefficiencies, and support better resource allocation when integrated effectively into production systems.
Every stage in the deployment process is critical to achieving reliable, efficient, and impactful results. With careful attention to data preparation, testing, and system integration, machine learning models can meet operational needs while adapting to future demands in manufacturing and other industries.
1. Data Preparation and Preprocessing
High-quality data is the foundation of any successful machine-learning model. This step involves collecting, cleaning, and preprocessing data to ensure it is relevant and consistent. Data preprocessing includes removing duplicates, handling missing values, and standardizing formats. Properly prepared data minimizes errors and enhances model accuracy, allowing robust predictions.
2. Model Development and Training
After data preparation, the next step is developing and training the machine learning model. This process involves selecting the right algorithms based on the specific use case, such as regression, classification, or clustering. The model is trained using historical data, learning patterns and relationships to make predictions or decisions. Training requires iterative adjustments to parameters to optimize model performance.
3. Model Validation and Testing
Validation and testing ensure the model performs accurately in scenarios. This step involves evaluating the model on a separate dataset not used during training, measuring accuracy, precision, and recall metrics. Robust testing helps identify potential weaknesses, ensuring the model is reliable and meets the required performance standards before deployment.
4. Integration into Production Systems
Once validated, the model is integrated into the production environment. This involves embedding the model into software systems, such as Manufacturing Execution Systems or enterprise platforms, where it can process live data. Integration also includes developing APIs or pipelines facilitating seamless communication between the model and existing systems.
5. Monitoring and Maintenance
Continuous monitoring ensures the model remains accurate and effective over time. Monitoring involves tracking performance metrics, identifying data drift, and addressing changes in production environments that might affect outcomes. Regular updates and retraining with new data keep the model relevant and aligned with operational needs.
6. Scalability and Optimization
Scaling the machine learning model to handle increased data volume or expanded operations is essential for long-term success. Optimization involves fine-tuning the model and infrastructure to ensure it operates efficiently as production requirements grow. Tools such as cloud-based platforms can support scalability while maintaining cost efficiency.
Machine learning deployment enables businesses to unlock the full potential of their data, enhancing production efficiency and enabling smarter decision-making. Clear processes for training, integration, and monitoring ensure models remain relevant and effective over time. These efforts help businesses achieve operational success and position themselves for continued growth in competitive manufacturing environments.
Challenges to Implementing AI in Production
Introducing artificial intelligence into production environments offers transformative potential but is not without its challenges. Manufacturers must address various obstacles to ensure AI systems integrate seamlessly, operate effectively, and deliver measurable benefits. These challenges often stem from data quality issues, resource limitations, and organizational resistance, requiring a well-structured approach to overcome.
Effectively navigating these barriers ensures AI-driven tools can improve efficiency, enhance decision-making, and contribute to long-term operational success.
- Data Quality and Availability: Poor-quality or incomplete historical data can undermine the accuracy of AI models during training and deployment. Inconsistent datasets limit the effectiveness of predictive tools, making high-quality data preparation essential for success.
- Integration with Existing Systems: Many manufacturers rely on legacy systems that are incompatible with AI technologies. Bridging this gap often requires substantial investment in infrastructure upgrades and time to establish seamless integration with modern AI tools.
- High Implementation Costs: While AI can reduce costs over time, the initial expenses associated with acquiring new technologies, hiring skilled personnel, and upgrading systems can be significant, particularly for smaller manufacturers.
- Workforce Resistance to Change: Employees may be hesitant to adopt AI-driven tools due to concerns about job displacement or unfamiliarity with new technologies. Resistance can hinder adoption and reduce the effectiveness of AI initiatives.
- Scalability Issues: Scaling AI tools to larger production lines or multi-site facilities demands robust infrastructure and clearly defined processes. Without careful planning, scaling efforts can create inefficiencies and resource constraints.
- Cybersecurity Risks: AI systems often rely on connected networks, making them vulnerable to data breaches and cyberattacks. Protecting production systems requires secure data handling, intellectual property safeguards, and continuous monitoring for threats.
Overcoming these challenges is essential for manufacturers aiming to realize the full potential of artificial intelligence. Addressing data quality, system integration, workforce readiness, and security concerns requires strategic planning and stakeholder collaboration. With the right approach, manufacturers can mitigate these risks while achieving the transformative benefits AI offers, including enhanced efficiency, cost savings, and operational scalability.
Measuring ROI of AI in Production
Evaluating the return on investment (ROI) of AI in production involves analyzing its contributions to efficiency, cost savings, and operational scalability. Metrics such as production throughput, defect rates, downtime reduction, and resource utilization highlight the measurable benefits AI delivers. Predictive maintenance reduces equipment downtime by identifying potential failures early, leading to cost savings and improved productivity. AI also enhances profitability over time through better resource allocation, lower energy consumption, and optimized inventory management, ensuring manufacturers can adapt to shifting demands effectively.
Qualitative benefits, such as improved product quality, faster time-to-market, and higher customer satisfaction, also play a critical role in ROI assessment. These factors strengthen brand loyalty and support long-term growth, even when they do not produce immediate financial returns. Combining both tangible and intangible results helps manufacturers fully understand AI’s value, empowering them to expand its role strategically and achieve sustained operational success.
Manufacturers worldwide embrace AI-driven solutions to enhance efficiency, precision, and scalability. At 42Q, we combine decades of manufacturing expertise with innovative cloud-based technology to deliver smart, connected manufacturing. Our flexible, cloud-native MES platform enhances visibility, streamlines operations, and accelerates digital congestion. Discover how our solutions can empower your factory to achieve its full potential.
Key Takeaways
- AI in production changes manufacturing processes through real-time optimization, predictive maintenance, and quality control.
- Machine learning adapts to production challenges using data-driven models that improve accuracy and efficiency over time.
- Implementing AI requires addressing data quality, system integration, and cybersecurity risks.
- Measuring ROI involves tracking quantitative metrics like defect rates and downtime reduction alongside qualitative benefits like customer satisfaction.
- Scalable and adaptable AI systems help manufacturers future-proof operations and respond to market requests.
FAQ's
AI in production refers to applying intelligent systems and algorithms to manufacturing processes. These technologies analyze data, identify patterns, and automate decision-making, helping manufacturers improve efficiency, optimize workflows, and reduce errors in real-time.
Machine learning improves production systems by allowing predictive maintenance, quality control, and request forecasting. It uses historical and real-time data to identify inefficiencies, detect anomalies, and adapt to conditions, making manufacturing operations more innovative and agile.
Industries such as automotive, medical devices, consumer electronics, and aerospace benefit significantly from AI production. These sectors rely on precision, scalability, and efficiency, all enhanced by AI-driven tools and processes.
The initial cost of deploying machine learning models in production can be substantial, covering technology acquisition, skilled personnel, and system upgrades. However, the long-term savings from optimized workflows, reduced downtime, and improved product quality often outweigh these upfront expenses.
Metrics like production throughput, defect rates, equipment downtime, and energy consumption are commonly used to measure AI’s impact. These indicators clearly show how AI contributes to cost savings, efficiency improvements, and operational scalability.